The DroneSOM project was started in spring 2022 to upgrade geophysical drone measurements and integration software and to combine these products to work together and have, better, more economically friendly exploration tools for mineral exploration.
Firstly, for data integration in 3D, Beak Consultants from Germany have developed advangeo® Prediction software. The software can now be used with different methods such as artificial neural networks (ANN) and self-organizing maps (SOM) methods for the prediction of spatial events and phenomena like the probability of geo-hazards or the location of mineral deposits. The software covers a complete workflow for predictions, including a collection of raw data, data preprocessing, and model parameterization. 3D visualization is made possible through a connection and interface to Paradigm GOCAD software. In addition, “advangeo® 2D Prediction” software existed already before the DroneSOM project, allowing 2D predictions and then visualization in Esri ArcGIS.
Secondly, for data integration in 2D, GTK from Finland has developed “GisSOM” software. The software generates a SOM from given multivariate input data and emphasizes visualizing the results in several ways in both the SOM reference frame and, in the case of spatial input data, the geographical reference frame. K-means clustering can optionally be applied to the SOM result, and the data distribution within each k-means cluster is visualized as scatter and boxplots as well. All the results are provided in numeric form as well so that the user can perform additional post-processing and visualization of the results if needed.
n the frame of the DroneSOM project, mineral predictive mapping using artificial intelligence with “advangeo® 2D Prediction” and “GisSOM” software was done using drone measurement data for a client, and it is presented here as a case study. The case study was conducted in 2023, using their existing data that included lithological and geochemical analysis, and tectonic structural studies. New data was collected with Radai’s drone, which collected magnetic responses of the subsurface in the study area (Figure 1). Also, GTK’s airborne electromagnetic and radiometric data and optional airborne magnetic data, lithological, structural, and geochemical data were used to help analyze and compare the results.
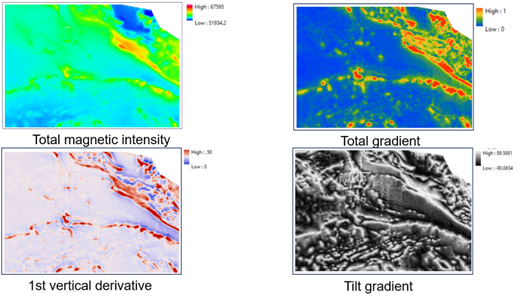
Figure 1 Radai’s collected drone-based magnetic data, processed data included tilt and 1st vertical derivatives.
Beak’s “advangeo® 2D Prediction” software was used to prepare several predictive maps based on available geophysical, geological, and geochemical (Au ≥ 2 ppm in outcrops) parameters within a training area and later outside the training area (Figure 2). The ANN maps are well influenced by the combination of the datasets used in the predictions and the accuracy and resolution are based on the quality of the available datasets. Therefore, choosing the training area and the quality of the dataset is important. In the ANN, the connection weights between neurons are the links between the inputs and the output signals and therefore are the links between the problem and the solution. Negative connection weight represents inhibitory effects on neurons (reducing the intensity of the incoming signal) and decreases the value of the predicted response, whereas positive connection weights represent excitatory effects on neurons (increasing the intensity of the incoming signal) and increase the value of the predicted response.
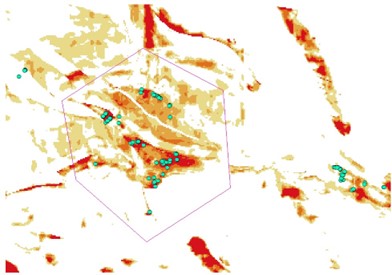
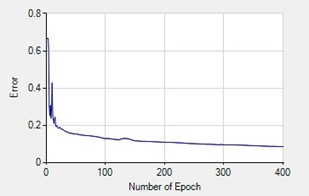
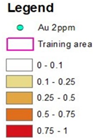
Figure 2. Predictions done with ANN are presented above. The model uses GTK radiometric (U, Th, K, and Total), resistivity, electromagnetic, Radai’s drone magnetic data and its derivatives, and fault length and direction with lithology of the area.
On the other hand, SOM analyses were done by GTK using the GisSOM software. The data sets were collected from ANN analysis and SOM K-means created 25 clusters for the geospacer, after this the high prospectivity classes were detected based on the known gold concentration in the area (Figure 3).
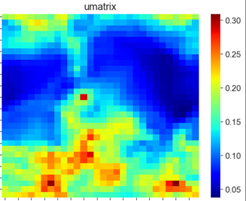
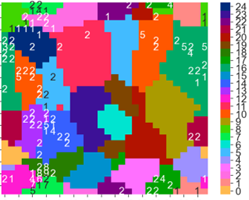
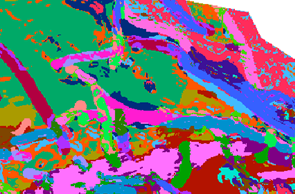
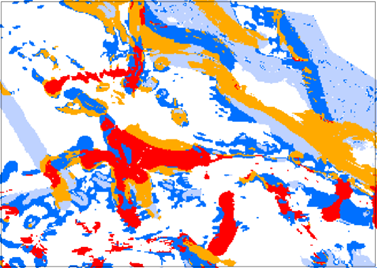
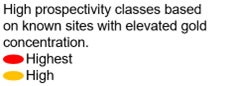
Figure 3. SOM analysis using GisSOM presenting the 25-cluster (on the top) used to detect the gold concentration in the area (at the bottom).
By comparing both ANN and SOM, the results are quite similar (Figure 4). Prospectivity mapping could work efficiently for the new exploration license area. ANN can be trained in nearby known areas that have good training data available. Trained ANN can then be applied to new exploration areas. Preparing a preliminary prospectivity map and comparing it with known occurrences is the key to getting good results and having new possibilities to expand the search areas. This maintains the focus on fieldwork in potential areas in the early exploration phase. Iterative work, repeat training, and modeling with additional field data and geological/geophysical/geochemical information once available will guarantee reliable results.
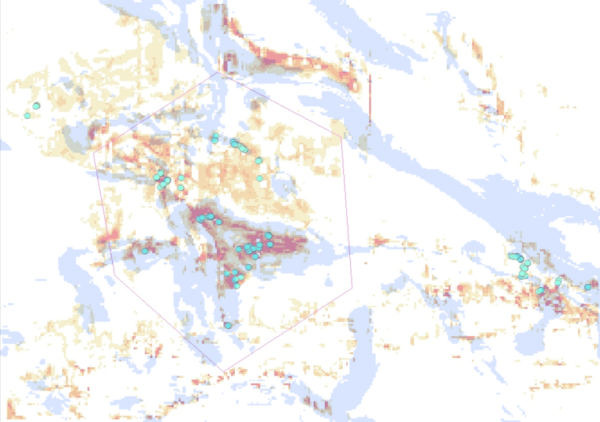
Figure 4. ANN prediction results on top of the SOM k-means cluster result. Present very promising similarities in the patterns.
Current developments by Beak in DroneSOM now focus to demonstrate mineral predictive mapping in 3D with another case study in the frame of the project using SOM. DroneSOM’s innovative approach, integrating geological parameters, regional geophysical data, and detailed magnetic drone surveys with advanced ANN predictive modeling and SOM technology, has demonstrated remarkable success in identifying promising and potentially mineralized areas. A groundbreaking project at the forefront of mineral exploration.